How to Teach Cybersecurity in the AI Era
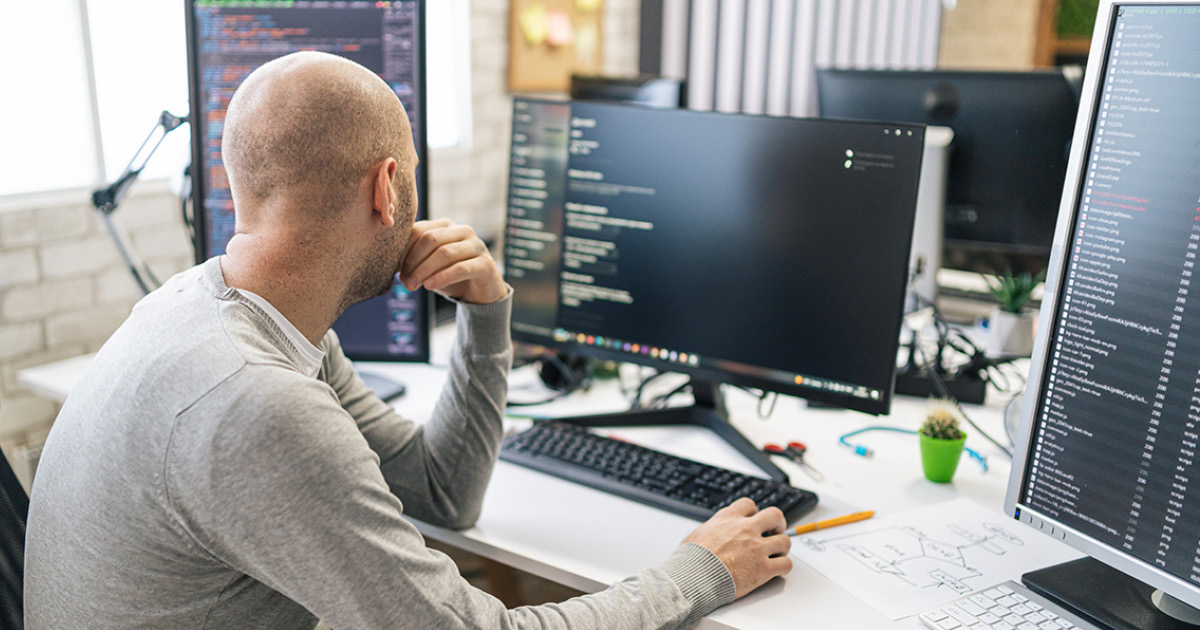
We can all agree that artificial intelligence (AI) has been one of the most talked about topics of the year so far. From a technology perspective, there are benefits and drawbacks to AI. For example, the benefits of artificial intelligence include the automation of mundane tasks that allow humans to become thought leaders and focus on process improvements and efficiencies. On the other hand, the drawbacks of artificial intelligence are very well publicized. For example, the potential for the elimination of jobs—300 million by one estimate—in various industries where tasks are repeatable and sequential (in some cases, mundane).
Additionally, there is also the risk of fraud, realistic voiceovers of recognizable people, deep fakes (realistic videos of recognizable people), and potential (sometimes unintended) bias. It is equally important to note that artificial intelligence tools are basically models that can be trained based on inputs and constant learning algorithms. These tools have helped hackers write malicious code as an example.
As cybersecurity educators, we must condition our students to continuously understand how to mitigate the inherent risk of artificial intelligence-related technology (continuously, because artificial intelligence is not going anywhere anytime soon) and ensure the confidentiality, integrity, and availability focal points of cybersecurity are emphasized. Read on to learn how to incorporate AI into your cybersecurity curriculum.
AI Requires Constant Calibration
In terms of calibration, let me paint a picture that we’ve seen at one point or another: a manufacturing assembly line. Imagine an assembly line that requires a device like a hammer that drives a nail into a specific section of a product to ensure that the product will remain intact once it is sold in the marketplace, and ultimately leveraged by a consumer. This assembly line can produce many products per hour, and the hammer device drives a nail into each and every product; it’s calibrated to drive a nail in a specific location day in and day out. Over time, it is safe to say that this hammer may begin to slightly drift from its specific impact location and the results may not appear until the product hits the marketplace; thus, resulting in a defective product or (even worse) injury. This is where the need for calibration comes into play.
From a manufacturing perspective, in terms of calibration, there’s a periodic (weekly, monthly, etc.) assessment of the hammer to ensure that it’s functioning as designed, and driving the nail in its precise location, with a minimal degree of variance. This is precisely the same approach that should be taken with artificial intelligence models. You see, without constant calibration, the results of the artificial intelligence models will be skewed, and defective.
Now, from a cybersecurity perspective, you can think of the analysis of log files that contain unauthorized attempts to access a network. Imagine if the analysis tool leverages artificial intelligence to distinguish legitimate, authorized attempts from cybersecurity threats and, over time, begins to identify false positives. The unnecessary research and resolution of false positives can take a toll on cybersecurity departments that are already stretched thin and fatigued. In this situation, consistent calibration can ensure that the analysis and results of the log files are accurate. This type of scenario can be applied to any cyber-related topic or process that incorporates artificial intelligence to detect and alert anomalies. It is safe to say there are risks with incorporating AI into cybersecurity, but calibration is imperative.
AI and The Human Element
As artificial intelligence models (and machine learning models for that matter) continue to run millions of processes, predictions, and decisions, calibration is crucial, and that is conducted by humans. Data is crucial to AI as it continuously learns based on inputs, but the data must be accurate. Without accurate data, the output from AI is useless: garbage in, garbage out.
Additionally, to ensure the AI tool doesn’t go off the rails, it is imperative to have human engagement incorporated within the AI process not only for calibration, but data integrity, and the prevention of bias. This is also known as human in the loop.
From a risk perspective, ensuring that AI decisions and output do not promote bias, human in the loop is a critical oversight control to ensure decisions made by the tool do not lead individuals, strategists, and executives down the wrong path.
It is important to inform your students that AI is here to stay, and it’s constantly getting better, smarter, and faster, but there will always be a need for humans to provide calibration and oversight. The only time that humans will not be needed to perform tasks is when the AI tools outnumber humans; we can control that. Also, as human educators, we can ensure that critical thinking and decision-making skills are instilled in students. Critical thinking and decision making will allow students to take an objective, holistic approach when solving problems; both of which are crucial non-technical skills for cybersecurity.
We have the power to enable the next generation of cyber professionals to ensure AI tools promote accuracy and fairness.
To learn more about how to teach AI, consider requesting a review copy of Fundamentals of Information Systems Security, Fourth Edition. This text includes information and discussion points on emerging technologies and the risks, threats, and vulnerabilities associated with our digital world.
Request Your Digital Review Copy
About the Author:
Rodney F. Davis is an adjunct professor at Syracuse University’s College of Professional Studies where he teaches courses focused on Enterprise Risk Management, Cybersecurity, Networking, Forensic Accounting (Fraud Prevention), and Vendor Risk Management. Rod has a total of 29 years professional experience, 27 of which are focused on operational risk, regulatory oversight, technology, and cyber security within the financial services industry. Rod is also a member of an international team of cyber risk professionals responsible for creating and approving certification exam items for ISACA (Information Systems Audit and Control Association).
Related Content:
- When It Comes to Cybersecurity, People are the Weakest Link—Unless We Educate Them
- Artificial Intelligence is Helping Hackers Write Malicious Code—Cybersecurity Educators Have a Role to Play to Stop Them
- Cybersecurity Students Want Real-World Experience in the Classroom. Here’s How to Give It to Them.